About Research
Understand the brain mechanism of decision making through machine learning
Our laboratory studies the circuitry of decision making in the dorsal cortices. Our aim is to understand how the brain generates complex behaviors by combining sensory inputs and prior knowledge. We are particularly interested in how the neuronal processes of decision making are functionally different from machine learning algorithms. Although recent artificial neural networks (or artificial intelligence: AI) achieve magnificent performance in visual processing, Shogi, Go, and StarCraft, there are still some tasks which are easy to solve for animals but difficult for AI. We use mice as a model system and combine behavioral tasks, calcium imaging, optogenetics, electrophysiology, and computations to address these questions.
My bachelor’s degree is in engineering, especially in robotics, from the University of Tokyo. At that time, before the era of deep learning, I got interested in neuroscience to understand how the brain accomplishes the sophisticated sensory processes and action selection. After I got a PhD in information science and technology, I continued my career in neuroscience with Dr. Kenji Doya at Okinawa Institute of Science and Technology (OIST) and learned interdisciplinary approaches of computational theory and experiment. At OIST, I investigated the neural substrate of dynamic Bayesian inference in the cerebral cortex. I then did my second postdoc with Dr. Anthony Zador at Cold Spring Harbor Laboratory (CSHL) and studied the neural substrate of perceptual decision making in the mouse auditory cortex.
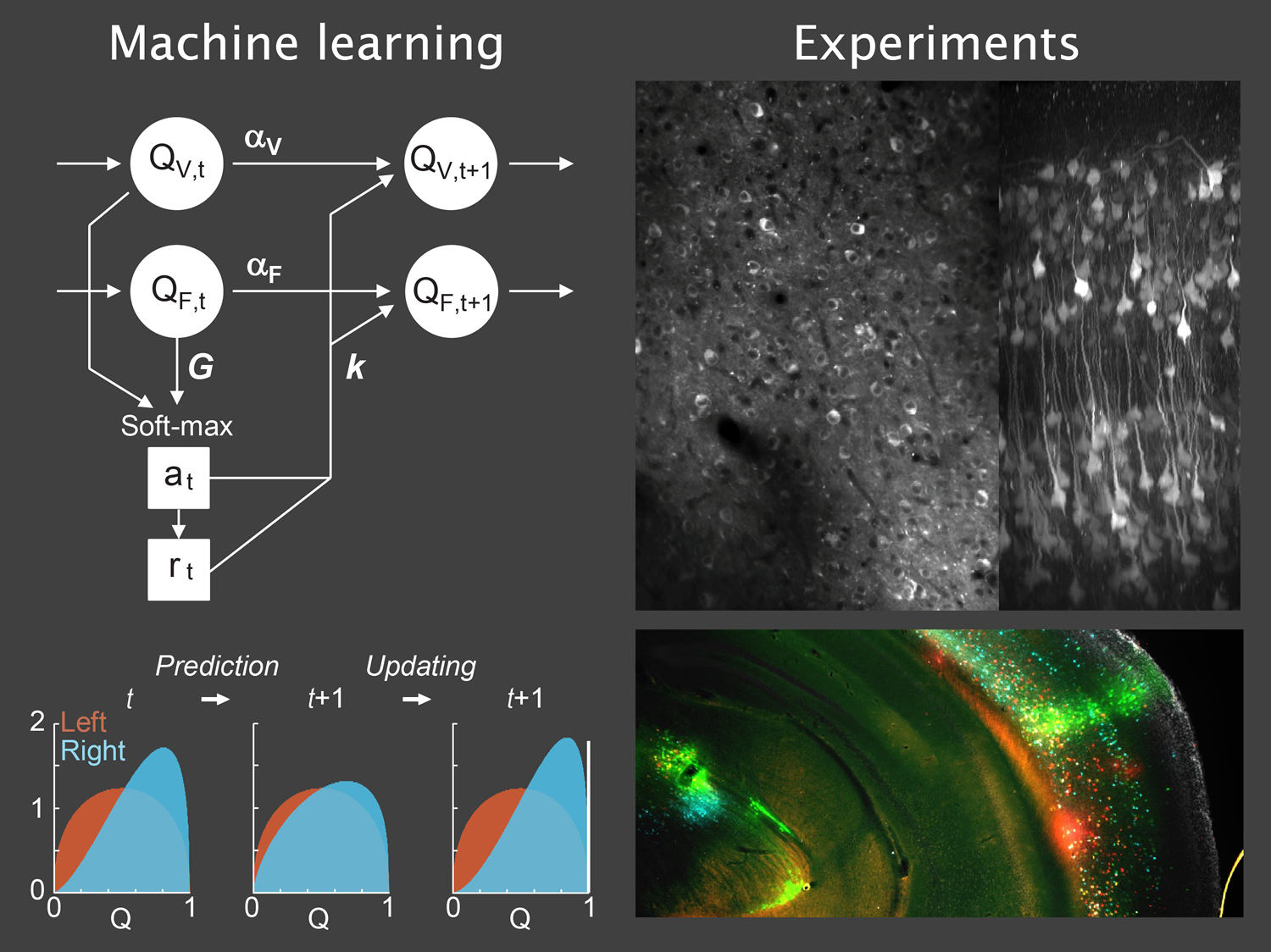